Readme
This documentation contains weekly assignments for the MPC-MAP course as well as the semester project assignment and a brief description of the simulating tool.
Please note that the documentation is continuously updated, the final version of the assignment will be available at the beginning of the given week. The same applies for the semester project – the assignment will be introduced by a lecturer during the semester.
Week 2 - Uncertainty
The goal of this assignment is to get familiar with the simulator, and explore uncertainties in the sensors and motion.
Task 1 – The simulator
Download the simulator from GitHub https://github.com/Robotics-BUT/MPC-MAP-Student, and get familiar with it (see the Simulator section). Focus your attention on the private_vars
, read_only_vars
and public_vars
data structures, and the sequence of operations in the infinite simulation loop. Try loading different maps and setting various motion commands. No output is required in this step.
Task 2 – Sensor uncertainty
The robot is equipped with 8-way LiDAR and GNSS receiver. Determine the standard deviation (std) sigma
for the data from both sensors by placing static robot (zero velocities) into suitable maps, and reading the data for at least 100 simulation periods. Discuss whether the std is consistent across the individual LiDAR channels, and across both GNSS axes. Plot histograms of the measurements.
Task 3 – Covariance matrix
Use the measurements from the previous step, and MATLAB internal cov
function to assemble covariance matrix for both sensors. Verify the resultant matrix is of size 8x8 and 2x2 for the LiDAR and GNSS, respectively. Verify the values on the main diagonal equal to sigma^2
, i.e. equal to variance=std^2
.
Task 4 – Normal distribution
Create a function norm_pdf
for assembling probability density function (pdf) of the normal distribution. The function accepts three arguments: x
(values at which to evaluate the pdf), mu
(mean), and sigma
(standard deviation). Utilize this function and sigma
values from the Task 2 (e.g. for the 1st LiDAR and X GNSS axis) to generate two pdfs illustrating the noise characteristics of the robots sensors, and plot it into one image (use mu=0
in both cases).
Task 5 – Motion uncertainty
The uncertainty is not just in the measurement (in the sensor data), but in the motion as well. Load the indoor_1
map and try to navigate the robot to the goal location without using sensors. To do so, apply a proper sequence of motion commands inside the plan_motion.m
function. Save the screenshot of the successful run and discuss, what could be the potential source of uncertainty in the robot motion.
Submission
To implement the tasks, use the algorithm
directory only; do not modify the rest of the simulator. The solution must work in the fresh simulator without any errors, and must generate the graphical outputs contained in the report.
Create a single A4 report that will briefly describe your solution (few sentences for each task and an image, if makes sense).
Send the report and zip
archive comprising the algorithm
directory to the lecturer's e-mail by Sunday 23:59 of the given week of the semester.
Week 3 - Motion control
This week, implement a simple path-following algorithm using motion capture-based localization data.
Make sure that your simulator version is up-to-date as the older versions do not provide MoCap.
Task 1 – Creating maps
Look into examples located in the maps
folder and try to identify meaning of individual parameters by means of the reverse engineering. Then, create an arbitrary custom map containing at least one wall. The map is going to be used to test your motion control algorithm so make it rather spacious. You need to define a GNSS-denied region to simulate indoor environment where the motion capture works.
Task 2 – Sample paths
Define at least three test paths of different kind, for example:
- straight line,
- circular arc,
- sine wave.
A path is defined as a sequence of (x, y) waypoints. Note that waypoints stored in the public_vars.path
variable are visualized by the simulator. Utilization of this variable is recommended.
Task 3 – Motion control
Choose any path-following algorithm and implement it to make the robot follow the paths defined in the previous task. Use the variable read_only_vars.mocap_pose
to get nearly true pose of the robot. Discuss how parameters of the chosen method affect the quality of the motion control.
Put your solution to the plan_motion
function. By default, the code structure is prepared for target point-based algorithms; you are, however, free to alter the function and use, e.g., the cross track error instead. The choice depends on your preferences, you can even implement multiple algorithms and compare them.
Mind the fact that the MoCap data will not be available for the final project!
Submission
To implement the tasks, use the algorithm
directory only, do not modify the rest of the simulator. The solution must work in the fresh simulator without any errors, and must generate the graphical outputs contained in the report
Create a single A4 report that will briefly describe your solution (few sentences for each task and an image, if makes sense).
Send the report and zip
archive comprising the algorithm
directory to the lecturer's e-mail by Sunday 23:59 of the given week of the semester.
Week 4 - Particle Filter
The goal of this assignment is to implement a working localization algorithm based on the particle filter.
Task 1 – Prediction
Implement the prediction function predict_pose
that takes the particle pose and the control input as arguments and returns the new pose. Apply the probabilistic motion model.
Task 2 – Correction
Implement the measurement function compute_lidar_measurement
that takes the map, the particle pose, and the lidar orientations as arguments and returns a vector of measured distances without any noise.
You may take advantage of the simulator function ray_cast
which is used as follows:
intersections = ray_cast(ray_origin, walls, direction)
The first argument is a position of the ray's initial point, the second argument is the description of walls stored in read_only_vars.map.walls
, and the final argument is a direction of
the ray in radians. The function returns all intersection of the given ray with the walls.
Tip: You can use the MoCap pose and lidar measurements to verify that your function returns correct data.
Then, implement the weighting function weight_particles
. You may use an arbitraty metric.
Task 3 – Resampling
Implement the resampling function resample_particles
, taking a set of particles and their associated weights as arguments and returning a new set of resampled particles. Use any algorithm of your choice.
Task 4 – Localization
Initialize a set of particles at random poses within the map. Update the particle filter in each iteration (perform prediction, correction and resampling). Adjust the previously implemented functions to make the particles converge to the robot's true pose. You should be able to present a screenshot with a cluster of particles gathered around the agent. You may need to move the robot in some cases to make the localization work.
Tip: Begin in a map with distinctive features, i.e. not containing similar corridors etc. You can create your own map to help you with the task.
Discuss the most important parameters of your solution and justify the selection of algorithms. What was the major issue you have had to overcome?
Submission
To implement the tasks, use the algorithm
directory only, do not modify the rest of the simulator. The solution must work in the fresh simulator without any errors, and must generate the graphical outputs contained in the report
Create a single A4 report that will briefly describe your solution (few sentences for each task and an image, if makes sense).
Send the report and zip
archive comprising the algorithm
directory to the lecturer's e-mail by Sunday 23:59 of the given week of the semester.
Week 5 - Kalman Filter and EKF
The goal of this assignment is to implement a localization algorithm based on the Extended Kalman Filter and GNSS data.
Task 1 – Preparation
Load the outdoor_1
map and manually design a trajectory between the start pose [2,2,π/2]
and the goal location [16,2]
.
Examine the uncertainty of the GNSS measurement; i.e. implement an initialization procedure which will determine the initial position (mean) and the covariance matrix of the GNSS sensor data. You will need the data within later tasks.
Task 2 – EKF implementation
As you use a differential wheeled robot, there is a nonlinearity in the state and control transition (nonlinear function g(x)); thus, you must employ the EKF for the prediction step. Implement ekf_predict
function for given drive type.
On the other hand, the KF is appropriate for the correction phase, since there is a linear relation between the state and measurement. Implement kf_correct
function.
Task 3 – Filter tuning
Use the known initial pose x=[2,2,π/2]
and Σ with zeros (i.e., high certainty) as the initial belief for the EKF algorithm, and navigate the robot along your trajectory to the goal location. Only the EKF-based estimated pose can be utilized for the control. You already know the GNSS covariance matrix to assemble the Q
matrix (measurement noise covariance); for the process noise, apply variances 0.01 (a rough initial estimate) to all variables and tune the R
matrix to reach an optimal performance. Capture the result.
Task 4 – Algorithm deployment
In practice, the true initial pose is not know for the algorithm. Utilize your initialization procedure from the Task 1 to determine the initial belief for the EKF. Notice that you can not measure the robot orientation, therefore a high variance must be specified for this variable within the initial belief.
Observe, how the estimated pose converges to the real pose values, and eventually tune the filter parameters to reach an optimal performance (a "smooth" estimate). Notice the orientation is successfully estimated even it is not directly measured. Capture a successful ride to the goal location.
Submission
To implement the tasks, use the algorithm
directory only; do not modify the rest of the simulator. The solution must work in the fresh simulator without any errors, and must generate the graphical outputs contained in the report.
Create a single A4 report that will briefly describe your solution (few sentences for each task and an image, if makes sense).
Send the report and zip
archive comprising the algorithm
directory to the lecturer's e-mail by Sunday 23:59 of the given week of the semester.
Week 6 - Path Planning
The goal of this assignment is to implement a working path planning algorithm.
Task 1
Choose and implement an algorithm which finds a path between the starting position of the robot and the goal position in the map. The path must not collide with any wall. Use the occupancy grid stored in the variable read_only_vars.discrete_map.map
.
Task 2
Adjust the planning algorithm to keep a clearance from obstacles. You may use any method. The clearance should be at least 0.3 m.
Task 3
Apply a smoothing algorithm to the generated paths. Use the iterative algorithm provided in the lecture, or find another. Discuss the effects of the algorithm's parameters.
Submission
To implement the tasks, use the algorithm
directory only, do not modify the rest of the simulator. The solution must work in the fresh simulator without any errors, and must generate the graphical outputs contained in the report
Create a single A4 report that will briefly describe your solution (few sentences for each task and an image, if makes sense).
Send the report and zip
archive comprising the algorithm
directory to the lecturer's e-mail by Sunday 23:59 of the given week of the semester.
Semester Project
Project objectives
The primary objective of the semester project is to develop a viable solution for the provided MATLAB simulator. Your code should guide the robot to its goal in the fewest possible iterations. The algorithms should be capable of working across various map types, and accommodate arbitrary goal and starting positions, including orientation. It is imperative that the robot avoids collisions with obstacles and remains within the boundaries of the map.
Successful completion of the project requires the ability to accomplish the task in all sample maps provided in the maps
folder. While suitable methods have been covered in lectures and assignments, you are not required to utilize every presented procedure. You are permitted to modify files within the algorithms
folder and add your own functions; however, altering the main.m
script is strictly prohibited. Note that step 7 of the simulator’s main loop will be disabled, rendering MoCap data unavailable.
Simulator
The simulator is accessible in the repository https://github.com/Robotics-BUT/MPC-MAP-Student. Do not modify any functions relied upon by the simulator; your solution will be evaluated using the functions published in the repository.
Your code must be executable without dependence on any MATLAB toolbox.
The solution will be assessed using MATLAB R2023b. The project must yield no errors upon execution.
Tip: Clone a 'clean' copy of the simulator from the repository and utilize a toolbox-free installation of MATLAB to ensure that your project encounters no issues.
Assessment
The project will undergo testing by the lecturers using various maps and randomly selected coordinates for the start and goal positions (including orientation). The success rate of reaching the goal and the required number of iterations will be statistically evaluated through repeated execution of the code.
Submission
Send the zip
archive comprising the algorithm
directory to e-mail address tomas.lazna@ceitec.vutbr.cz by Sunday 23:59 of the 8th week of the semester (31 March 2024).
Scoring system
- Success rate of reaching the goal: up to 15 points
- The required number of iterations: up to 15 points
- Technical quality: up to 20 points
To successfully pass the course, a minimum of 20 points must be obtained from the semester project.
MATLAB Robot Simulator
The simulator is lightweight, MATLAB-based tool for testing key algorithms utilized for autonomous navigation in mobile robotics. Basically, it integrates differential drive mobile robot model equipped with two different sensors (lidar and GNSS), and enables to deploy it within custom 2D maps. The main goal is to navigate the robot from start to goal position; for this reason, several algorithms must be implemented:
- Localization: two algorithms are needed - for outddor and indoor. The pose may be estimated via Extended Kalman Filter and GNSS data in outdoor areas; for indoor, the algorithm utilizing Particle Filter and known map is more suitable since there is a GNSS denied zone indoor.
- Path planning: an algorithm to find optimal, obstacle-free path from the start to goal location (e.g. A* and Dijkstra's algorithms).
- Motion control: a control strategy to follow the computed path by using the actual estimated pose. This results in the control commands for the individual wheels.
Variables
The simulator uses numerous variables to provide its function; however, not all of them can be used/red to solve the task (the robot's true position, for example). The variables are divided into three groups (structures):
- Private variables (
private_vars
): these variables are used in the main script only and not accessible in modifiable student functions. - Read only variables (
read_only_vars
): these are accessible for your code, but not returned to the main script. - Public variables (
public_vars
): feel free to use and modify these variables and add new items to the structure. The majority of student functions return the structure to the main script so you can use it to share variables between the functions.
Except these variable structures, other variables can occur in your workspace, there is no limit to their use.
Simulation Loop
The simulator workspace comprise main script stored in main.m
, which contains the main simulator logic/loop and is used to run the simulation (F5
key). After the initialization part (you are expected to modify the setup.m
file called in the beginning), there is a while true
infinity simulation loop with the following components:
- Check goal: check if goal has been reached.
- Check collision: check whether the robot has not hit the wall.
- Check presence: check whether the robot has not left the arena.
- Check particles: check the particle limit.
- Lidar measurement: read the lidar data and save them into the
read_only_vars
structure. - GNSS measurement: read the GNSS data and save them into the
read_only_vars
structure. - MoCap measurement: read the reference pose from a motion capture system and save it into the
read_only_vars
structure. - Initialization procedure: by default, it is called in the first iteration only; used to init filters and other tasks performed only once.
- Update particle filter: modifies the set of particles used in your particle filter algorithm.
- Update Kalman filter: modifies the mean and variance used in your Kalman filter algorithm.
- Estimate pose: use the filters outputs to acquire the estimate.
- Path planning: returns the result of your path planning algorithm.
- Plan motion: returns the result of your motion control algorithm. Save the result into the
motion_vector
variable ([v_right, v_left]
). - Move robot: physically moves the robot according the
motion_vector
control variable. - GUI rendering: render the simulator state in a Figure window.
- Increment counter: modifies read-only variable
counter
to record the number of finished iterations.
Steps 8 to 13 are located in a separate student_workspace.m
function.
Warning! Step 7 is going to be skipped during the final project evaluation. Your solution must not rely on MoCap data!
You should be able to complete all the assignments witnout modifying the main.m
file.
Custom Functions
You are welcome to add as many custom functions in the algorithms folder as you like; however, try to follow the proposed folder structure (e.g., put the Kalman filter-related functions in the kalman_filter folder). You may also arbitrarily modify the content (not header) of the student workspace function (steps 8 to 13 of the simulation loop) and other functions called by it.
Maps and testing
The maps
directory contains several maps in the text file format, which are parsed in real-time when the simulation is started. Use reverse engineering to understand the syntax, and create own maps to test your algorithms thoroughly. In general, the syntax includes the definition of the goal position, map dimensions, wall positions, and GNSS-denied polygons. Do not forget to test various start poses as well (including start angle!); this is adjustable via start_position
variable (setup.m
). For the project evaluation, a comprehensive map comprising both indoor and outdoor areas and GNSS-denied zones will be employed.
GUI
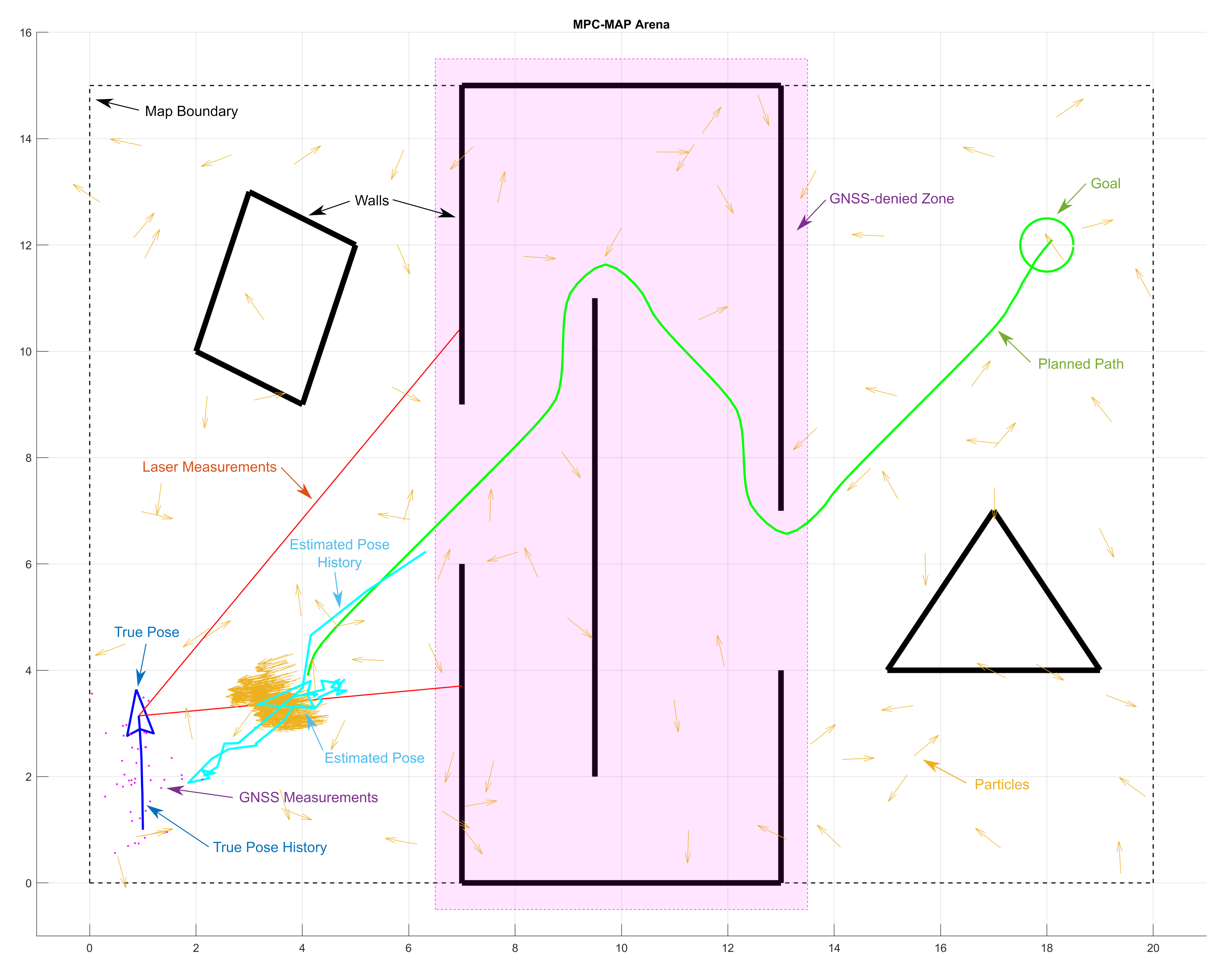